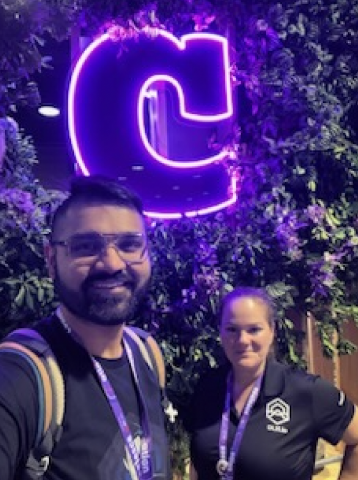
As we were headed towards day 3- We were halfway through, but still, a lot to cover, and the schedule had so many sessions planned for the day. The day-3 program was primarily divided across the below categories:
- Perspective altering/Architectural Point of view
- Scaling and automation solutions
- Hands-on Labs
- Customer use cases/ dbt applications in real world
Quick highlight of some of the key sessions.
Money, Python, and the Holy Grail: Designing Operational Data Models
What do analysts are hired to do? Building dashboard? Data pulls or clean up messy data? Or dabble with millions of pitch decks. The answer is probably a little obvious: to perform impactful, strategic analysis agnostic to the data stack or underlying technological setup. In this talk, Benn Stancil (Mode) emphasizes the value of frameworks and going back to the drawing board to focus on fundamentals when the complex ideas/analysis do not stack up.
This beautiful reference to the IS-LM model curve from Paul Krugman’s blog post/ analysis of the past 2008 financial crash economy emphasizes the power of basic frameworks doing the work when complex calculations break down.
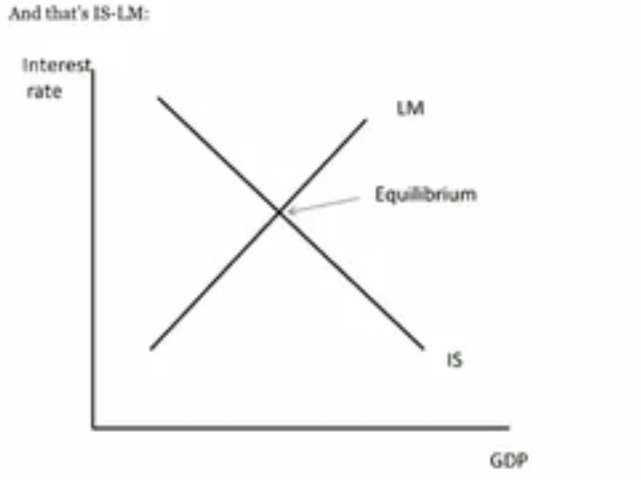
From Data Magician to Data Coach
The talk started with a powerful statement – 80% of analytics insights will not deliver outcomes. This is an important point, as more often than not – the analytics are insightful, but the outcomes/decisions might not match up and hence the need to change the lens from a data magician and think about data coaching to ensure that everyone works together to solve the problem at hand.
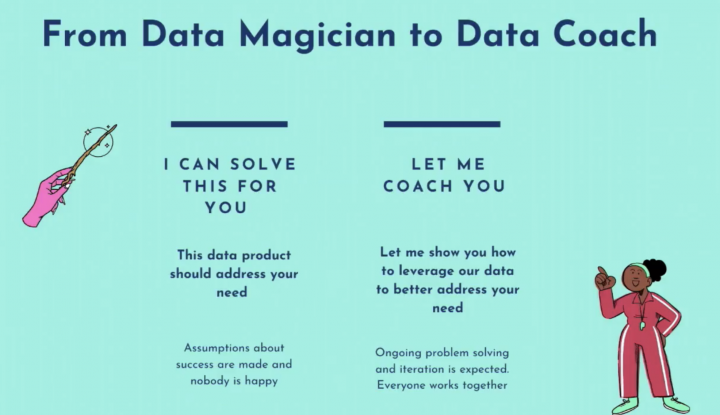
Hands-on: the dbt Semantic Layer
Remember the pain of endless discussions/interpretations of the metrics in use? Many times, the data is correct, and the calculations are accurate. The problem is the interpretation of the metric. Why leave the metric definition subjective and standardize it, so the entire organization speaks the same language? This is where the concept of the dbt Semantic Layer helps. By defining metrics centrally in dbt, data teams can trust that business logic referenced anywhere will be the same everywhere.
Lets just end this section with one thought from the session – Today’s metrics lead into tomorrow’s semantics.
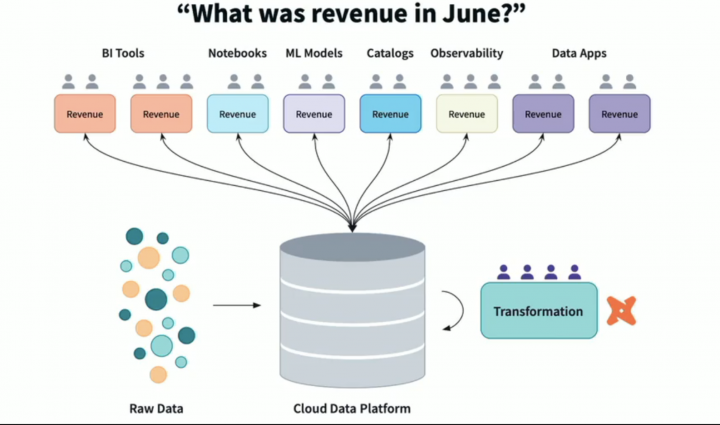
Announcing dbt’s Second Language: When and Why We Turn to Python
The session makes you think – When is it helpful to use Python, and when should you stick with SQL instead? For the first time in dbt, you can now run Python models, making it possible to supplement the accessibility of SQL with a new level of power and flexibility.
Various scenarios were demonstrated where Python does better than SQL and vice-versa but concludes with the idea that they work better together in dbt. This is an exciting development as the dbt-py models, in turn, lead to a host of possibilities in the future – supporting MLOPs within the dbt ecosystem and reaching a certain level of language agnosticism, killing the debate of Python vs. SQL vs. R.
Operational AI for the Modern Stack
“MLOPS is drowning in Complexity”
Firms have spent the last few years trying to emulate Uber’s state-of-the-art ML setup, which is very complex due to the number of services and components! This stack makes sense but is only for a limited number of organizations, and setting up a massive/complicated setup leads to infrastructure, team, and Operational challenges.
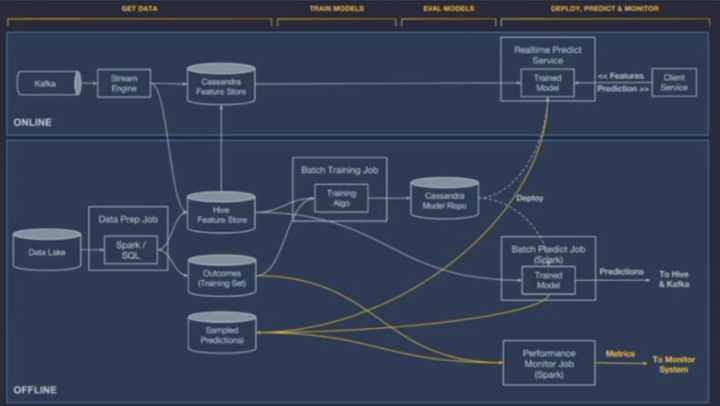
So what’s the solution? – The talk touches upon the use of dbt and Continual to scale operational AI and simply the architecture to meet the goals of current ML/AI needs.
Vendor Stalls
Day-3 was opening day for the vendor booth offerings and hosted a variety of vendors across the modern data stack
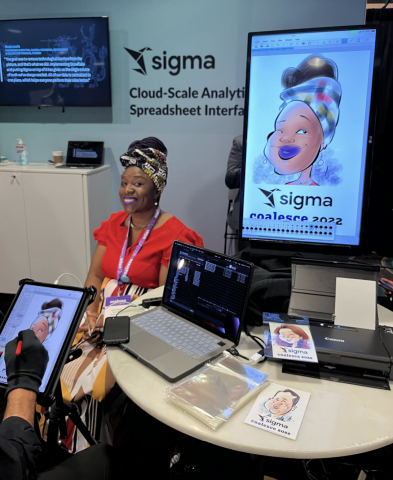
The day concluded in a similar boisterous way with various after-party sessions starting at 6pm. More details on the after parties to come!